As AI adoption grows, businesses must weigh performance versus cost when selecting large language models (LLMs). Galileo’s Agent Leaderboard (https://huggingface.co/spaces/galileo-ai/agent-leaderboard) provides a data-driven approach to this comparison, ranking AI models based on efficiency, accuracy, and pricing. Recent evaluations highlight that while performance differences among top models remain small, cost variations can be significant—impacting decisions around budget, scalability, and total cost of ownership.
The table below illustrates the current leaderboard ranking, showing each model’s position, vendor, cost per million tokens (I/O), and average category score (TSQ). Notice how the top three models score closely in performance, yet their costs vary significantly.
Analysis of these leading LLMs shows that top-performing models can differ in accuracy by only 2%, yet their pricing varies by 10x. Some open-source models deliver results on par with proprietary alternatives, reducing dependency on costly API-based solutions. Additionally, computational demands for high-end models contribute to increased operational costs, making cost-performance trade-offs essential for real-world deployments.
In the chart below, each dot represents a model, plotted by performance score on the vertical axis and cost per million tokens on the horizontal axis. Despite minimal gaps in performance among the top models, the cost difference can be substantial. This visualization highlights why budget and use-case requirements should factor heavily into model selection.
Beyond accuracy, efficiency metrics—such as processing speed and resource consumption—play a key role in determining a model’s cost-effectiveness. Some models prioritize low-latency performance and are better suited for real-time applications, while others focus on maximizing accuracy and accept higher computational costs in return. These trade-offs directly influence how a business scales AI-driven solutions.
Choosing the right LLM requires evaluating not just raw performance but also operational efficiency, resource requirements, and total cost of ownership (TCO). As AI adoption expands, balancing accuracy, price, and scalability remains a central challenge in model selection.
Tismo helps enterprises leverage AI agents to improve their business. We create LLM and generative AI-based applications that connect to organizational data to accelerate our customers’ digital transformation. To learn more about Tismo, please visit https://tismo.ai/.
You May Also Like
These Related Stories
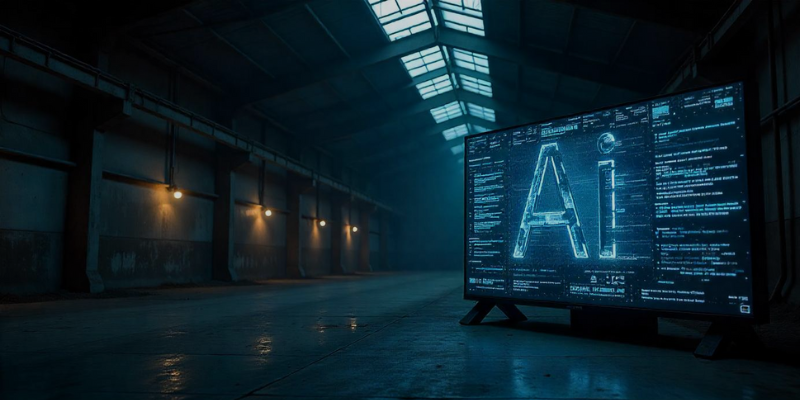
Prompt Engineering Increasing Relevance
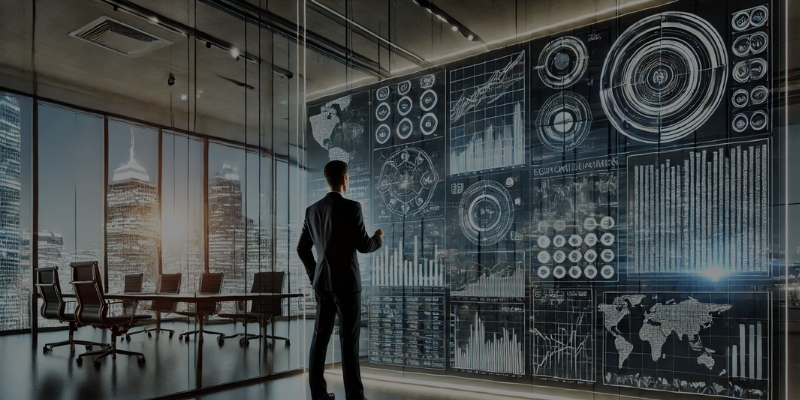
Agent Evals Compared to LLM-as-a-Judge
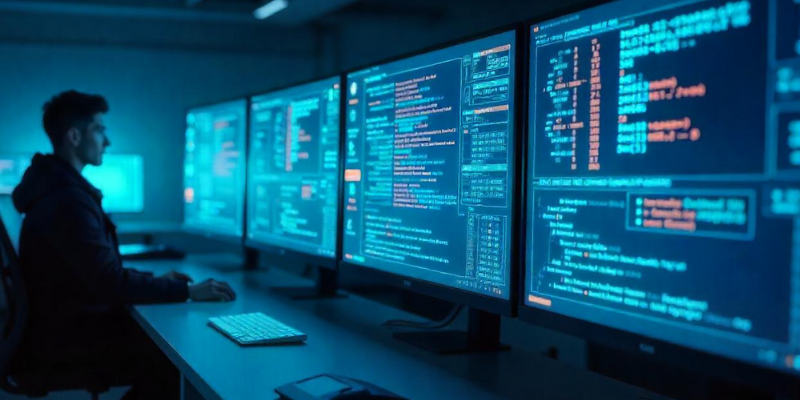